Potential fraud is a consideration for every brand with a referral program. Inevitably, someone will attempt to test the system by referring themselves.
Your Advocate program has an optional, automated Fraud Protection feature that can be used together with manual actions like blocking email domains and specific participants and IP addresses to mitigate the effects of fraudulent behavior. However, keep in mind that the strongest defense against referral program fraud is effective program design.
Referrals are only placed into a pending state after you turn on Fraud Protection for your Advocate account.
From the left navigation menu, select
[Menu] → Settings.
From the Advocate Settings section, select Security.
Select
[Toggle on] Automatic fraud detection.
When Fraud Protection is turned on, Advocate will evaluate and assign a fraud score to all new referrals.
The fraud score ranges from 0 (no risk detected) to 100 (very high risk). It is calculated by comparing each new referral against previous referrals to look for similarities. The overall score assigned to a referral determines whether it’s automatically approved or denied, or placed into a pending state for manual review.
Factor | Influence on score | Example Referral Information |
---|---|---|
Same email address | High | |
Same name (exact match only) | High | Robin Banks and Robin Banks |
Same IP address | Medium | 192.158.1.38 and 192.158.1.38 |
Similar email address | Medium | |
Similar name | Low Only used to support email address and IP address detectors. | ROBIN BANKS and Robby Banks |
Advocate supports three Fraud Protection levels: Relaxed, Moderate, or Strict. The level you select applies to all of your referral programs. Legacy referrals won’t be evaluated.
The Relaxed setting is best for brands who want to streamline their referral program and reduce the likelihood of denying legitimate referrals. This level allows more referrals while accepting the possibility of some self-referrals.
Using the Relaxed setting, most referrals are automatically approved. If a referral is highly likely to be fraudulent, then it will be automatically denied.
Fraud Score | Action |
---|---|
0 to 84 | Automatically approved |
85 to 100 | Automatically denied |
The Moderate setting is appropriate for brands with capacity for hands-on review of their referrals. This level provides a middle ground, allowing manual review of potential self-referrals for a more accurate referral program.
Fraud Score | Action |
---|---|
0 to 69 | Automatically approved |
70 to 84 | Manual review required |
85 to 100 | Automatically denied |
Typically, programs using instant access widgets immediately grant a reward to the referred friend. When Fraud Protection is turned on and Advocate detects a suspicious referral attempt, the referral is either denied or placed into a pending state, depending on its fraud score and your settings. Customer advocates see a message within the widget letting them know the referral was either denied or flagged for manual review. For pending referrals, no reward is issued unless you approve the referral.
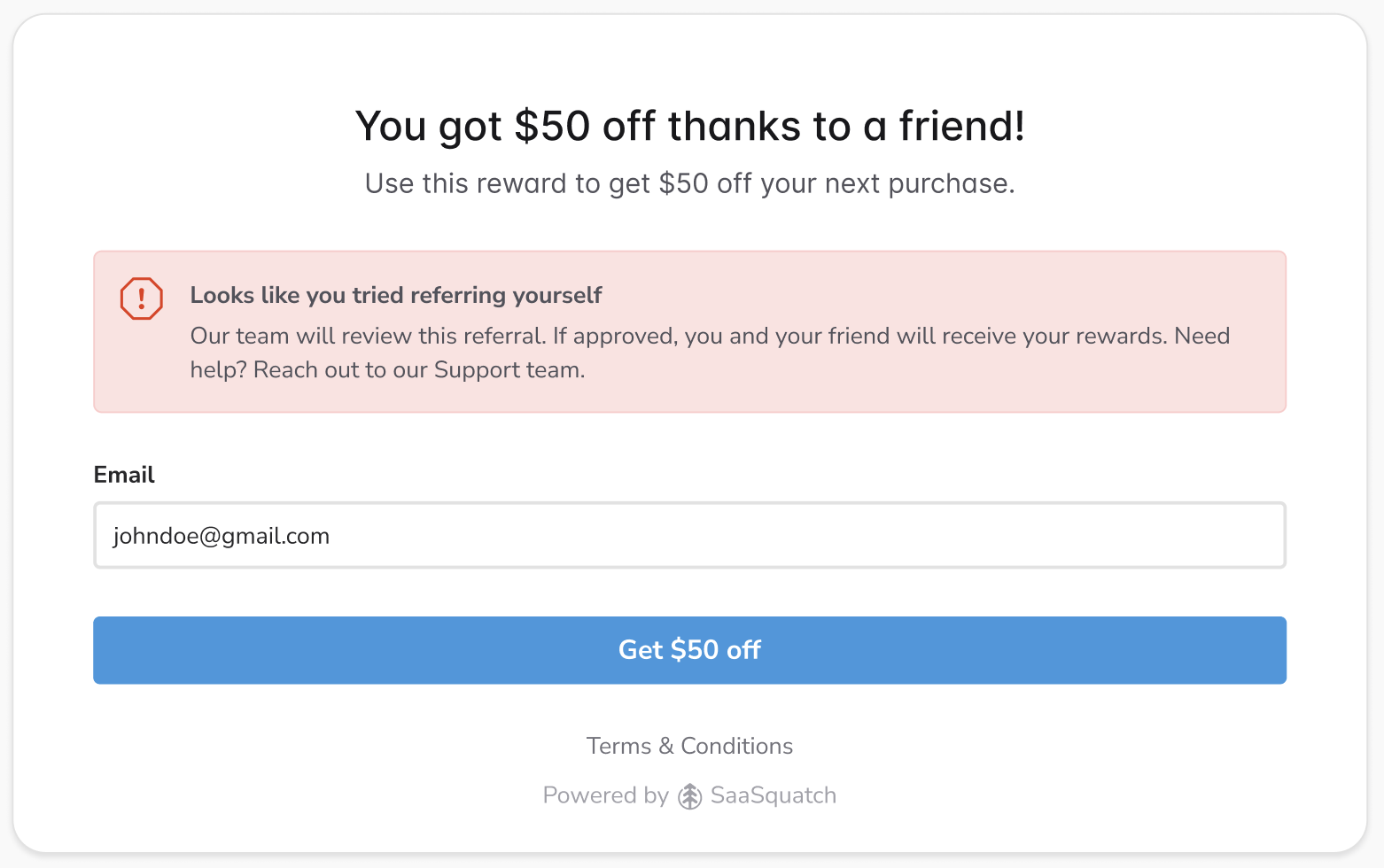